In recent years, the adoption of AI in law has revolutionized the way firms handle laborious tasks such as document review, case analysis, legal research, etc. However, a concern amongst lawyers remains: AI Hallucination.
What is AI Hallucination?
In simple terms, AI hallucination occurs when a model generates information that sounds plausible but is factually inaccurate or unfounded. This issue arises when AI systems make confident assertions that aren’t grounded in reality, often due to gaps in training data or limitations in their retrieval mechanisms. Hallucinations pose unique risks in legal AI applications, where precision, reliability, and adherence to legal precedents and regulations are paramount.
Why Does AI Hallucination Occur?
Understanding the causes of hallucinations is critical to building trustworthy AI systems. Factors like data quality issues, the limitations of language models (LMs), and the complexity of language generation tasks can all contribute.
Here are five ways to help prevent AI hallucination and ensure the reliability of legal AI tools.
1. Optimize Training Data and Knowledge Bases
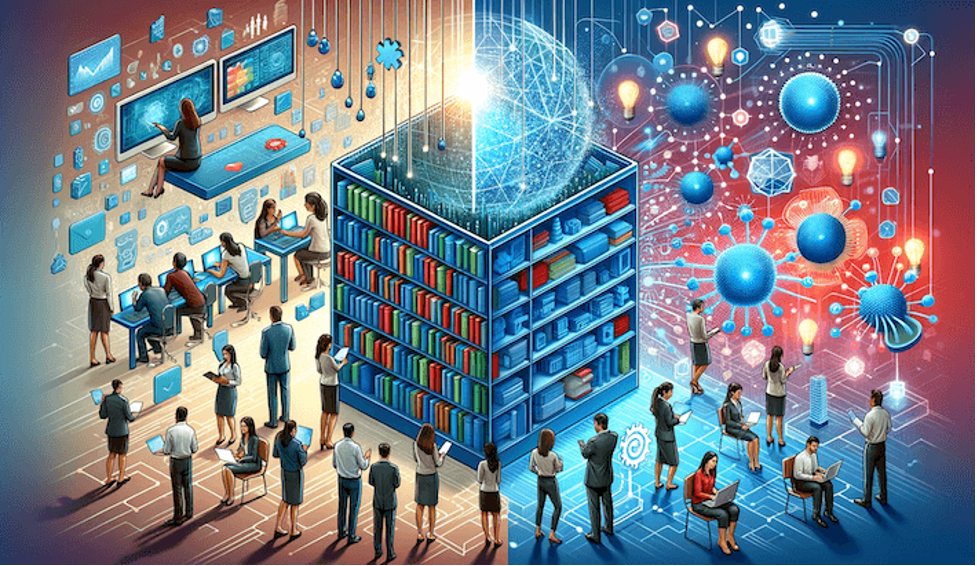
To reduce the risk of hallucination, it’s crucial to start with high-quality data and a well-structured knowledge base.
- Create a Knowledge Base
Build a comprehensive knowledge base containing FAQs, case precedents, statutes, and other legal resources relevant to your clients. Organize this information in a way that allows the AI to sort through it accurately and retrieve relevant data when generating responses. A robust knowledge base grounds AI responses in verified information.
- Train or Fine-Tune on High-Quality Data
When training legal AI models, prioritize quality over sheer quantity. A smaller, high-quality dataset can yield better results than a large, unstructured one. Ensure that your data covers a balanced array of legal contexts to minimize biases and enhance model understanding. Regular fine-tuning with recent, relevant data also reduces the likelihood of hallucination.
- Clean Your Data
Data cleaning is essential. Remove duplicates, outdated information, and irrelevant content before feeding data into the model. This “data detox” prevents hallucinations and improves model performance by ensuring only reliable information is accessed.
- Test and Select Reliable Large Language Models (LLMs)
Testing various LLMs helps identify which models offer the most reliability in high-stakes legal contexts, where speed, accuracy, and scalability are essential.
2. Implement Robust Verification Systems
Verification and filtering mechanisms ensure that your AI’s outputs remain accurate and aligned with your knowledge base.
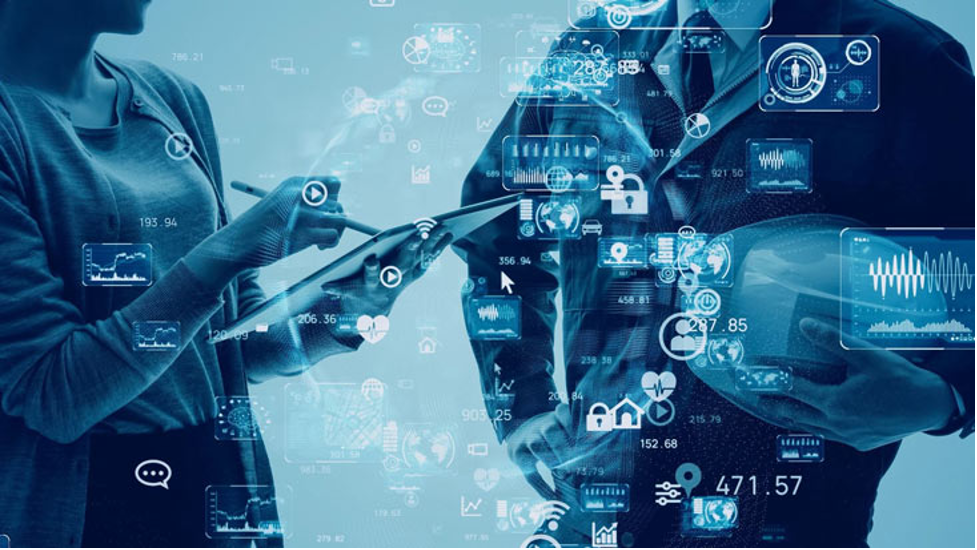
- Establish Verification Checks
Implement checks that verify AI-generated responses against the original knowledge base to confirm accuracy and relevance. Comparing AI outputs with verified documents prevents inaccurate information from reaching end-users.
- Regularly Update the Knowledge Base
Keep the knowledge base current with the latest legal precedents and regulatory changes to prevent outdated information from causing hallucinations. This ensures the AI has a solid, up-to-date foundation of legal knowledge.
3. Use Retrieval-Augmented Generation (RAG) for Real-Time Access
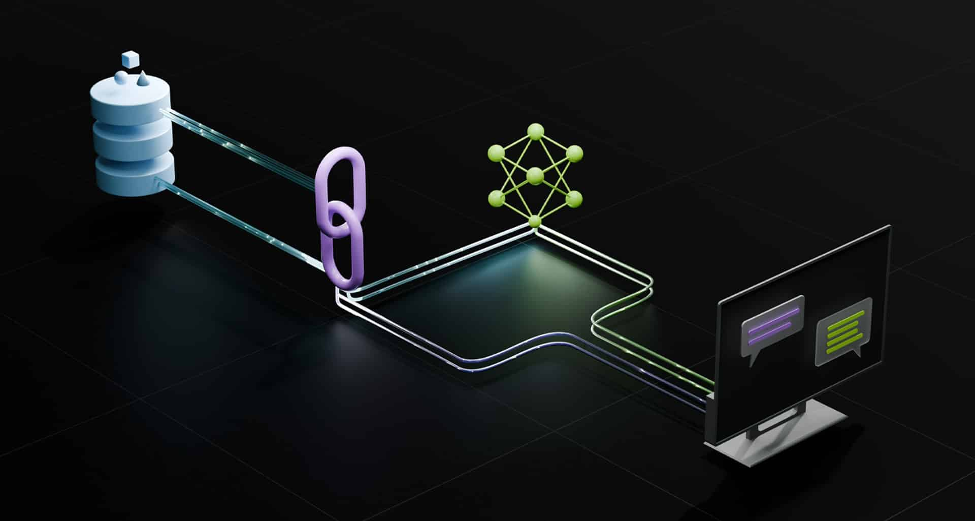
Retrieval-Augmented Generation (RAG) combines retrieval and generation capabilities, allowing AI to access real-time information from trusted databases instead of relying solely on pre-trained knowledge.
- How RAG Prevents Hallucination
RAG enables legal AI tools to retrieve current statutes, case law, and recent amendments as needed, grounding responses in the latest legal developments. This real-time access reduces hallucination and enhances the AI’s factual accuracy.
4. Define Clear AI Roles and Limitations
AI models are more prone to hallucination when tasked with broad or undefined roles. Define clear parameters for what the AI can and cannot do within a legal setting.
- Establish Defined AI Boundaries
Assign specific tasks to the AI, such as contract review, or legal research. Providing clear boundaries helps prevent speculative responses outside its scope. Additionally, set up monitoring systems that flag responses outside these defined tasks for review.
5. Implement Ongoing Human-AI Collaboration
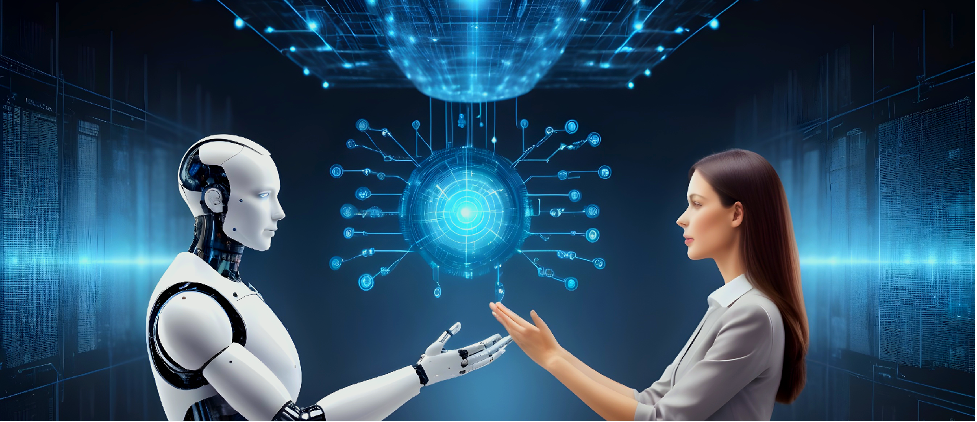
Human oversight is critical in preventing hallucination, ensuring the accuracy and relevance of AI outputs.
- Encourage Feedback Loops
Enable legal professionals to review AI outputs and provide feedback, creating a continuous improvement loop. This real-world feedback helps the AI refine its accuracy, reducing hallucination risks over time.
Conclusion
Preventing hallucination in legal AI demands a combination of high-quality data, rigorous verification protocols, advanced technologies like RAG, and consistent human oversight. By implementing these key strategies, the legal industry can develop reliable, fact-based AI tools that effectively minimize the risks of AI-generated misinformation.